AI – power considerations
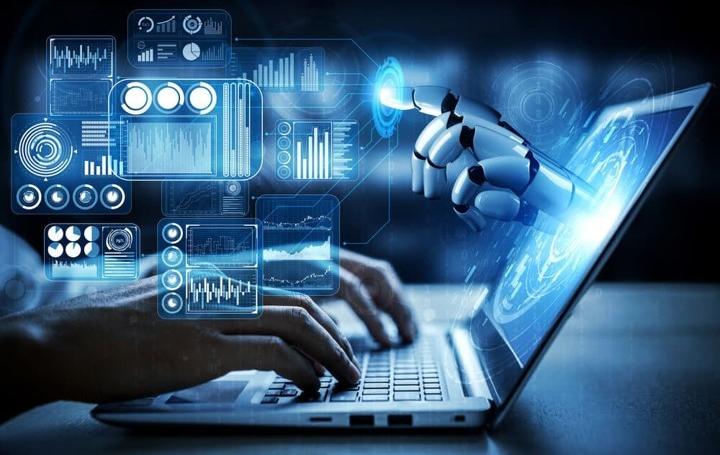
There has been much debate about what AI might evolve into in the future – an AI summit in the UK in November 2023 drew technology leaders from around the world to discuss possible outcomes, good and bad. Fittingly, the meeting was held at Bletchley Park, home to code breakers during the Second World War using the early computer ‘Colossus’, perhaps a precursor to the AI of today with its 2500 tubes, 17 square meter footprint, 5 metric ton weight and 8 kW power consumption. The ‘Turing test’ [1] to determine if a computer can ‘think’ is named after the Bletchley Park codebreaker Alan Turing and it is argued that the ‘Large Language Model’ ChatGPT now passes with ease.
AI limits - energy and hardware
So, what are the controlling influences on AI? Ethical considerations apart, there are practical limits in play, not least of which is the hardware involved and providing power to run it. Before AI, data centers showed huge increases in throughput to meet demands for services like video streaming and cloud-gaming, but the hardware kept up and developments in programming and power delivery system efficiency resulted in barely increased overall data center energy draw. For example, consumption rose by just 20% between 2015 and 2022 according to the International Energy Agency (IEA) [2]. This is despite a 340% increase in data center workload between the same dates.
Data centers, AI and energy – the numbers
Things are changing however with AI – the training phase of a Large Language Model (LLM) is energy intensive, ChatGPT reportedly consuming over 1.2 TWh. However, in the operational or ‘inference’ phase, the figure is around 564 MWh per day using nearly 30,000 NVIDIA GPUs in over 3,600 servers [3]. As a result of figures like these and all the other server applications, the IEA states that growth of data center energy use is now at a rate of 20-40% annually. Company Schneider [4] estimates that the AI CAGR element is 25% to 30% to 2028.
Rack power density is also increasing
Not only will power levels increase, but power dissipated per rack will also balloon – it has been determined that rack power density requirements are three times higher for AI applications than traditional ones. A graph from the Open Compute Project summit in 2023 (below) shows typically 4 kW in 2010 to commonly around 13 kW in 2023, but sometimes as high as 30 kW, with an exponential trajectory that could take it to hundreds of kW per rack in coming years.
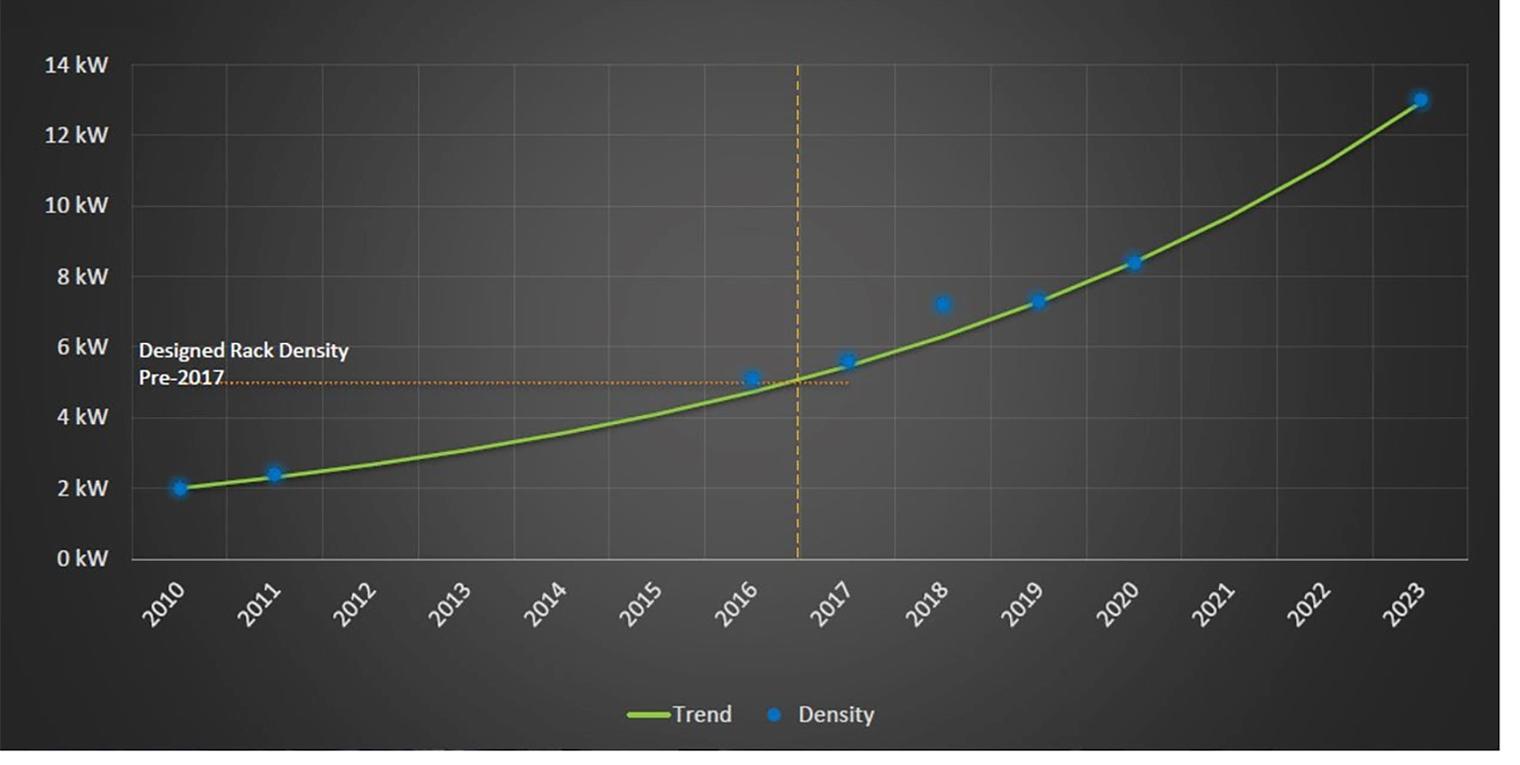
Increasing computing and hence power density in a rack is driven by the need to minimize data path lengths and to utilize floorspace to the maximum. This then provides the basis for capacity planning and sizing of cooling systems and critical power protection in the power distribution system.
Power distribution systems for AI hardware
There is a whole history of power distribution arrangements for IT equipment that have been used over the years. Now, as power levels have increased, along with a reduction in chip supply voltages, the accepted arrangement is to generate intermediate buses at relatively high voltages to keep currents low and distribute these around the cabinets and racks. Distribution at around 400 V is becoming popular around backplanes with appropriate safety considerations and this would be followed by DC/DC converters producing buses traditionally at isolated 48 V then later 12 VDC, with the lower value allowing higher conversion efficiency down to the final voltage, often sub-1 V, using Point of Load converters (PoLs).
Board-level distribution voltage is shifting back to 48 V
There is a move now back to 48 V as the current at 12 V has become so high that dissipation and volt-drop in connections are excessive. In the same connections, operation at 48 V reduces current to a quarter and dissipation to one sixteenth, so the gains are valuable. This is also enabled by new converter designs that allow high-efficiency down-conversion from 48 V to low voltages. It is also accepted that the 48 V bus need not necessarily be isolated at the board level, again allowing higher efficiency conversion. The PoLs close to the end-loads provide the final precisely regulated voltage necessary and this is electrically ideal, but if they are next to the load on the circuit board providing ‘lateral’ power, this can restrict access for data connections. Additionally, this puts the converter next to a component that might be dissipating 100s of watts with limited possibilities to extract heat from the DC/DC itself.
Working round the tough environment – ‘vertical power’ solutions
To find a workable solution, DC/DC manufacturers such as Flex Power Modules have come up with innovative solutions and one is the concept of ‘Vertical Power Delivery’ (VPD) [5]. Here, the PoL DC/DC is matched to the pin-out of a particular GPU or similar chip and fitted directly opposite on the underside of the board with top-side cooling. Being very low profile, the PoL does not affect board spacing and allows separate heatsinking while not blocking access for data lines and relying on the board traces for heat dissipation. PoLs capable of 1000 A peak and higher can be implemented this way.
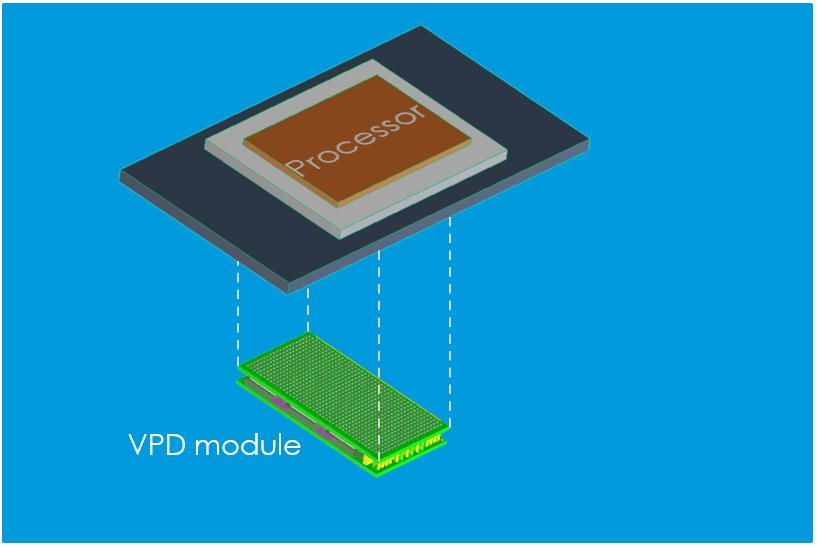
VPD is typically a custom solution, but for one that is not tied to a particular processor pin-out, lateral power can be implemented with standard off the shelf modules such as the BMR510 and BMR511 integrated power stages [6], which separate the power and control stages, with the power stage being a standard module, and the control implemented using discrete components. This minimizes the footprint of the PoL element close to the target load and is suitable for mid to high current rails, up to 160 A per module.
Will AI accept its own limits?
Perhaps one day, AI implemented in silicon will be able to suggest a solution to its own power consumption problems – or it may just conclude that with its spectacular creativity and intelligence, consuming around 12 W, the human brain can’t be matched.
This blog was not written by AI... or was it?
References
[1] https://en.wikipedia.org/wiki/...
[2] https://www.iea.org/energy-system/buildings/data-centres-and-data-transmission-networks
[3] Alex de Vries, The growing energy footprint of artificial intelligence, Joule, Volume 7, Issue 10, 2023, Pages 2191-2194, ISSN 2542-4351, https://doi.org/10.1016/j.joule.2023.09.004
[4] Avelar, Donovan, Lin, Torell, Torres Arango. Schneider white paper 110 v2, The AI Disruption: Challenges and Guidance for Data Center Design, https://www.se.com/ww/en/insig...
[5] https://flexpowermodules.com/scaling-new-heights-with-vertical-power-delivery
[6] https://flexpowermodules.com/top-and-bottom-cooling-options-for-integrated-power-stages